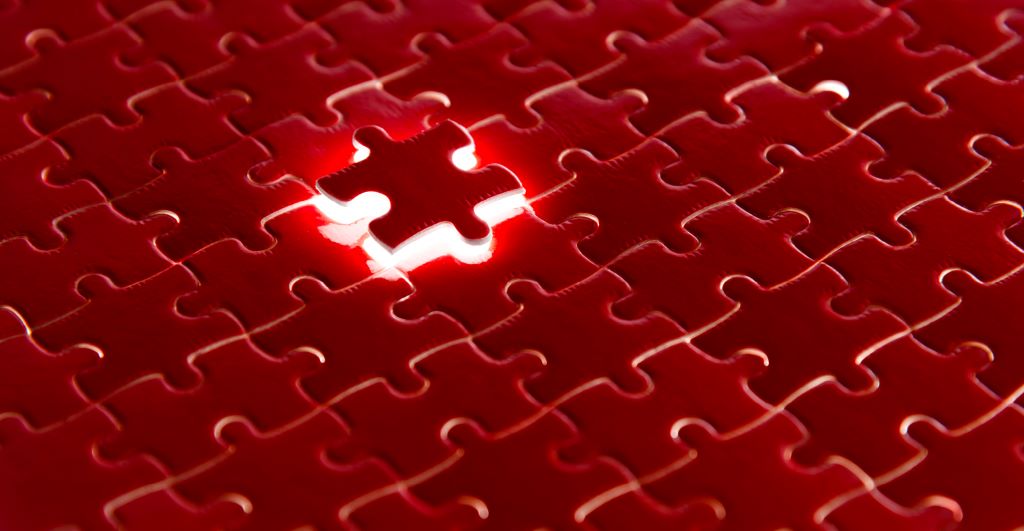
The introduction of Artificial Intelligence (AI) in networking has taken a well-worn path to market adoption. In early development, the theoretical possibilities are explored. We ask the big questions: Is this possible? What will it take? What are the applications? What are the implications? During this period, some ideas die on the vine. A few, however, take root as vendors, OEMs, and industry alliances begin exploring them in earnest. If there is enough interest and activity, the hype starts to build. Eventually, all the theoretical vetting must give way to implementation, iteration, and refinement.
Every major technology follows this path to market adoption. This includes 5G, SDN, Open ROADM, O-RAN and, most recently, Artificial Intelligence in its various forms. When Fujitsu started focusing on AI, Machine Learning (ML), and Generative AI, there was a lot of hype in the industry. We’ve moved past that initial phase and we are getting down to the realities of implementation, particularly as it applies to open networking. As an industry, we are learning a lot about what is possible. We’ve identified several strong use cases for AI in networking and we have a better understanding of the obstacles to implementation. The following is taken from my recent interview with Monica Paolini of Senza Fili. It provides an overview of what Fujitsu has learned about AI. You can read the entire interview transcript here.
Putting the technologies in context
If you haven’t been following the development of AI, ML, and GenAI, these three technologies can seem muddled. So, before we dive into the lessons learned, it may be helpful to quickly explain the relationships between AI, ML, and GenAI.
Machine Learning (ML) is the brains of the process. ML starts with raw data and uses statistical analysis, neural networks, and classification models to derive information.
Artificial Intelligence (AI) is the muscle. Armed with a variety of algorithms and policies, AI crunches the ML analysis and offers recommendations regarding actions to be taken or decisions that need to be made.
Generative AI (GenAI) is all about content creation. It receives input from the ML processes and AI recommendations to generate completely new ideas or content. While this may suggest tight integration between the three technologies, this isn’t always the case. Each can be deployed as a standalone solution where the data inputs come from a variety of sources. In fact, this is what we’re seeing in many of the most popular use cases.
Emerging use cases
In the telecom space, wireless and transport networks are growing more complex as the next generation enters the workforce, while network traffic and costs keep growing. AI-enabled automation can help operators address these changes. One example of where we’re seeing this is in the deployment, commissioning, and turn-up of new nodes in the optical transport network. In the provisioning stage, automation can be used to acquire the initial configuration of a new node, then confirm it is operating properly, and monitor the Key Performance Indicators (KPIs). Once the node is online, you can automate aspects of Day 2 operations.
Another emerging application is digital twinning where we build a digital mirror of the network to enhance planning and design. Fujitsu uses AI to update and analyze the network model in the digital twin. When changes to the network are implemented, we also use AI to monitor the performance, update the model, and predict outcomes for different scenarios.
Energy efficiency as well as its impact on the network and end user are other areas where AI, ML, GenAI, and automation are playing a significant role. These applications rely on the ability to run huge data sets and then consider the various trade-offs. For example, you may allow energy savings to have some impact on the quality of service for some users but not for others. This approach gives service providers the flexibility to optimize performance and power savings based on the use case and the user.
Specific use cases for GenAI include chat interfaces that have started to improve customer experience and operations. Troubleshooting a network issue, for example, often involves going through multiple technical documents from a variety of vendors to diagnose the issue. GenAI can use Large Language Models (LLMs) to quickly index all this data and offer an informed solution.
Adoption will occur gradually, but collaboration is key
It is going to be an iterative, step-by-step adoption process with network operators taking their time to develop confidence in the technology. This is especially true in telecom where operators tend to be risk averse. Initially, we may start with just analysis, where you can do a lot without directly impacting the network. You are not relying on a machine to provision your network, for example. But you can use ML to derive insights from that data. The models are improving with every iteration.
In the meantime, operators are watching cautiously to figure out where the technology can add value. They’re putting an air gap between the technology and their network. For now, at least, there will be a human who is going to process the output and filter out hallucinations.
Until now, much of the development has been occurring in silos. Moving forward means stepping up the amount of collaboration across the industry. Vendors must be actively engaged in customer lab trials and collaborate with partners and other vendors. We are now at a point where we need to begin implementing what we’ve learned, then iterating and refining.
This has already begun in earnest at Fujitsu where operators interested in our work in open networking are inviting us into their labs to evaluate our software and discuss our efforts in automating O-RAN, RIC, and SMO. We then bring in more vendors and introduce more interface points. This is how it starts, and then it gets moving quickly. Finally, integrators will continue to play a huge part in creating an environment for combining components and validating the system’s performance. We’re seeing this in Open RAN, but there needs to be more. Operators and service providers must encourage the ecosystem players to move towards openness. Automation through testing and integration will accelerate the process. Once we have the interfaces set and the platforms in place, the applications on top will bring value and extract more benefits from automation, whether by improving the network’s efficiency or delivering a new service to the end user.
We’re just getting started
There are still some significant challenges to overcome, including developing confidence in the technology, improving collaboration efforts, plus vetting our assumptions and data models. But, given the momentum behind AI, ML, and GenAI, we are well past the point of asking, “is it feasible?”
As we move deeper into the implementation phase, we are confirming the practical applications of AI/ML. Moreover, we are getting a better sense of its practical importance, such as the ability to solve specific problems and quickly generate quantifiable value for the operator. The evolutionary work we are doing now opens the door for more revolutionary implementations in the future. At Fujitsu and across the networking world, we’re just getting started.
For a deeper dive into how AI networking is transforming networks
Read my entire interview with Monica Paolini of Senza Fili