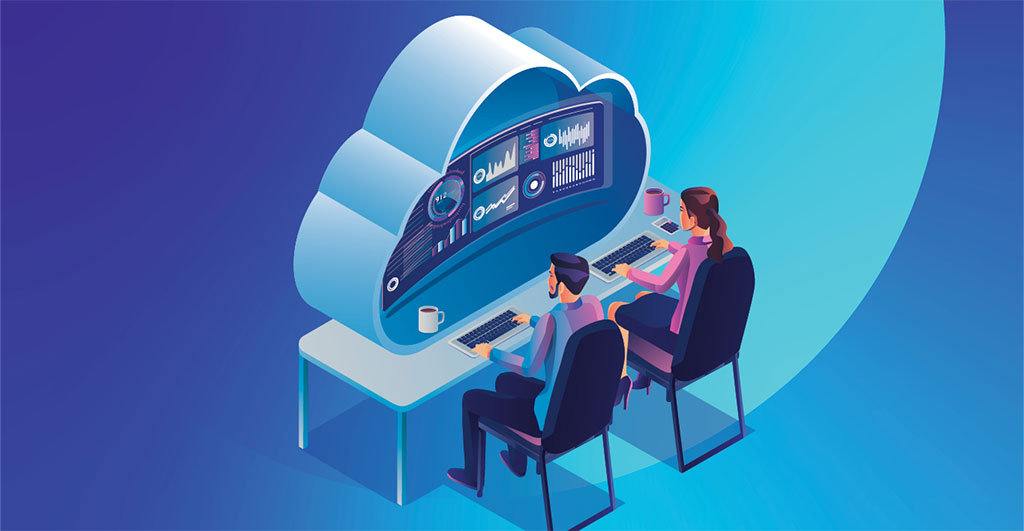
AIOps, abbreviated from “AI for network operations,” is becoming the evolutionary juggernaut of network transformation. Variants of AI technologies promise broadly stated value-adds, such as reducing complexity and boosting operational efficiency, but in order to realize value, it’s essential to cut through the hype and focus on real, achievable benefits as balanced against the time, effort, and cost of implementation.
AIOps: the coming foundation for network operations
So says Heavy Reading’s recent white paper, AIOps: The Value of Prediction , which assesses the evolution, adoption, benefits, and challenges of AI within the communications network industry at large. There’s definite consensus that AI tools are a necessity for transitioning to the new era of big data analytics and automated operations. Customer service expectations have evolved in recent years, and service requirements are now much more dynamic than in the past. Network operators no longer have the option of waiting for something to break and then fixing it; instead, they must find ways of predicting problems and taking evasive, rather than corrective, action to prevent service disruption from occurring at all. Eventually, AIOps will be the foundation of network operations—and the secret of success is to understand the cost-benefit relationship and thoughtfully prepare to overcome any implementation challenges.
Ever-stricter service KPIs & cost-reduction pressures are key drivers
The financial pressures facing network operators are twofold. On the one hand, stricter service quality requirements mean that they must guarantee higher performance standards just to prevent loss of revenue from customers switching providers or demanding compensation in the event of outages. On the other hand, capex and opex budgets are subject to ongoing downward pressure in terms of “doing more with less.” Done right, AIOps has the potential to deliver necessary service quality improvements while enabling reductions in both capex and opex. The big question is, what does “done right” mean?
Assessing implementation challenges
AIOps challenges fall into five categories, each of which merits careful consideration in relation to an individual operator’s network, business model, goals, and financial profile:
- Network: Data quality and harmonization can be costly and time consuming, but is essential to ensure accurate AI output and decision-making. Integration requires time and effort, but open, well-proven solutions can help move things forward efficiently. In some cases, specialized Large-Language Models (LLMs) may be needed to supplement the lack of specificity inherent to generic tools.
- Technology: Complexity means AI decisions must be carefully validated to ensure they are comprehensible, accurate, appropriately contextualized, and free from introduced bias—not to mention useful and practicable.
- Regulation: Regulatory frameworks are still evolving, and operators must work to ensure reliable data governance, validation criteria, and security measures.
- Business: AI tools and their integration carry a significant price tag and ROI timescales can be hard to assess, especially with still-maturing technology. Similarly, the need to source skilled professionals must be balanced against opex savings from eliminating repetitive, redundant tasks. Operators will also need to explore lower cost compute resources and energy-efficiency techniques (likely enabled by AI).
- Operational: Identifying skills gaps and adjusting operations structure towards AI goals is vital to successful implementation and maintenance of AIOps tools. Adapting AI to function within the telecommunications/communications network environment may require focused effort, especially in brownfield networks.
AIOps integration paths & autonomy levels
Specific AIOps use cases, integration goals and pathways vary by domain in today’s disaggregated, multilayer, multidomain, cloud-native networks; RAN, transport, core and edge domains perform different functions and are subject to different use case priorities. RAN operators, for instance, may focus on energy efficiency due to the significant energy consumption with radios, whereas core and edge teams are likely to focus on reducing mean time to repair to maintain primary route optimization.
That said, every domain has one factor in common: network data, which is essentially the lifeblood of AIOps. The first order of business is ensuring that network data is valid and free from noise or duplication, and that AI tools and LLMs are properly trained to use it. This may involve multiple approaches, including prompt engineering, retrieval augmented generation, and supervised fine-tuning.
Moving through autonomy levels
While network operators are motivated to introduce AI and network automation, the complexity and risk involved means that in most cases, they will implement progressively though a series of autonomy levels to move from manual operation though assisted operations, partial- and conditional-operations, eventually reaching high levels of autonomy as available tools, skills, and frameworks evolve and mature. Thus, it’s essential to view AIOps implementation as an ongoing, longer term process.
De-risking AIOps in a cautious environment
To a degree, adopting AI requires a certain amount of risk tolerance. But because the communications network industry is highly regulated and tends to be cautious, this means exploring risk via “safe mechanisms” that take into account multiple factors including interoperability, trustworthiness, openness, and the culture/philosophy and skills of operations teams.
Bridging legacy & future technologies & operations
Greenfield networks undoubtedly offer a “clean-slate” approach to AIOps implementation, but many if not most operators are taking their first steps in a brownfield environment where multiple network generations must coexist. These environments require careful attention to business and technical goals as well as to stakeholder buy-in, migration planning, and the potential for system consolidation.
AIOps is critical to staying competitive
Eventually, AIOps will extend across the full range of network operations as deployment scale and performance/quality demands increase. Timeframes will vary from operator to operator, depending on their specific goals and constraints, but there is little doubt that transitioning to AI, typically starting out with simpler, repetitive tasks, is a necessity for remaining competitive. While implementation is a long-term process, ultimately the resultant ability to predict and prevent will be worth the investment.
Heavy Reading’s white paper provides extensive detail about the evolution, benefits, and challenges of AIOps in the communications network industry, including discussion of specific use cases, tools, and integration considerations.